Blocking In Experimental Design Crunching The Data

Blocking In Experimental Design Crunching The Data Here are the main steps you need to take in order to implement blocking in your experimental design. 1. choose your blocking factor (s) the first step of implementing blocking is deciding what variables you need to balance across your treatment groups. we will call these blocking factors. Multivariate experiments. multivariate experiments are a great tool that can be used when you want to test multiple changes to an experience that are likely to interact with one another. this design allows you to test many changes at once, rather than sequentially testing individual changes. switchback experiments.
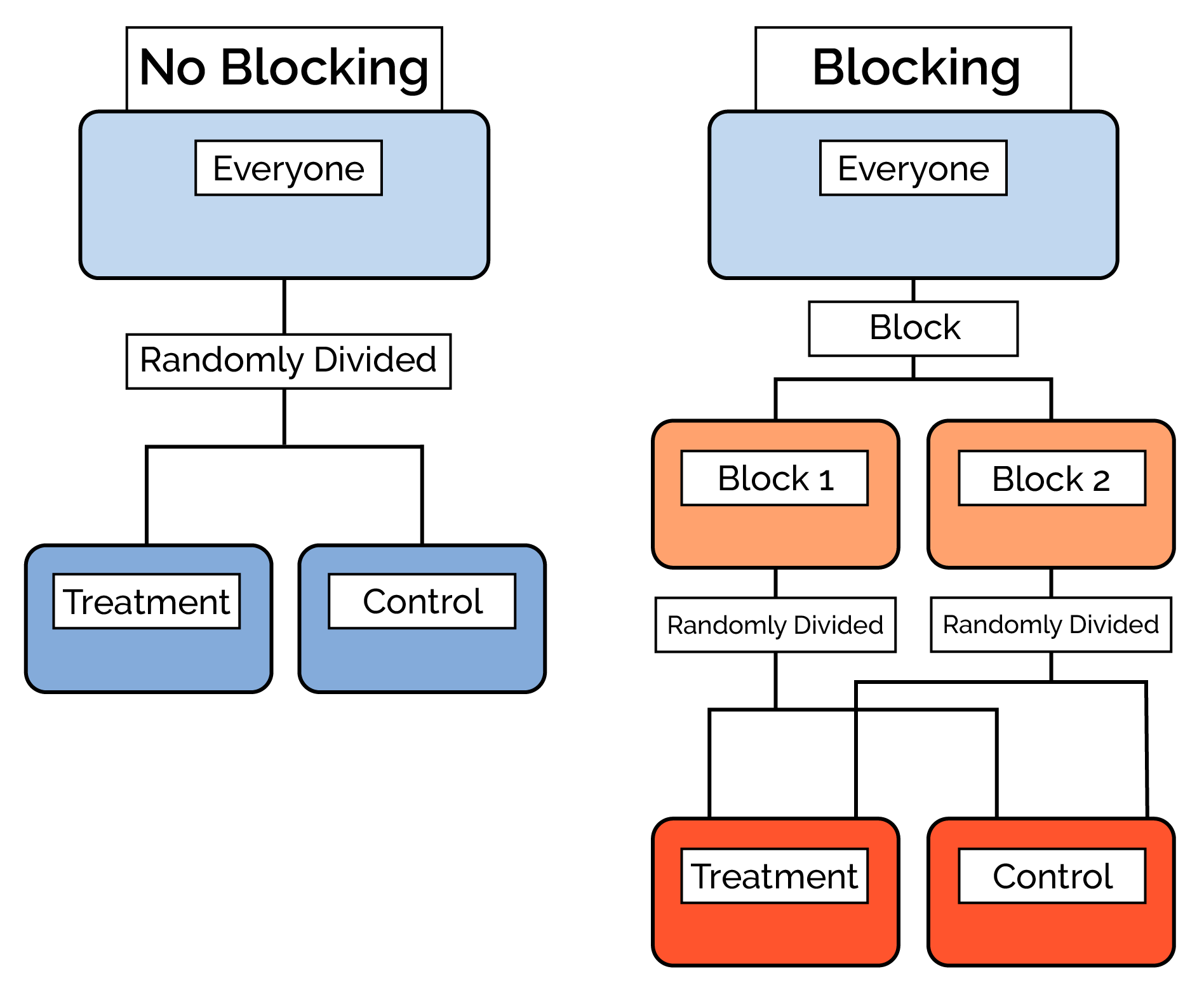
Experimental Design And Blocking Data Science Discovery Notice the two way structure of the experiment. here we have four blocks and within each of these blocks is a random assignment of the tips within each block. we are primarily interested in testing the equality of treatment means, but now we have the ability to remove the variability associated with the nuisance factor (the blocks) through the grouping of the experimental units prior to having. Objectives. upon successful completion of this lesson, you should be able to understand: concept of blocking in design of experiment. dealing with missing data cases in randomized complete block design. application of latin square designs in presence of two nuisance factors. application of graeco latin square design in presence of three. Blocking first, then randomizing ensures that the treatment and control group are balanced with regard to the variables blocked on. if you think a variable could influence the response, you should block on that variable. a diagram showing an external design using no blocking (left side) vs. blocking (right side). The general idea behind cuped is that when you analyze your experiment, you can supplement the data that you collected during your experiment with additional data that was collected before the start of the experiment. in doing so, you can reduce the variance of the coefficient estimates in your experiment and effectively reduce the sample size.
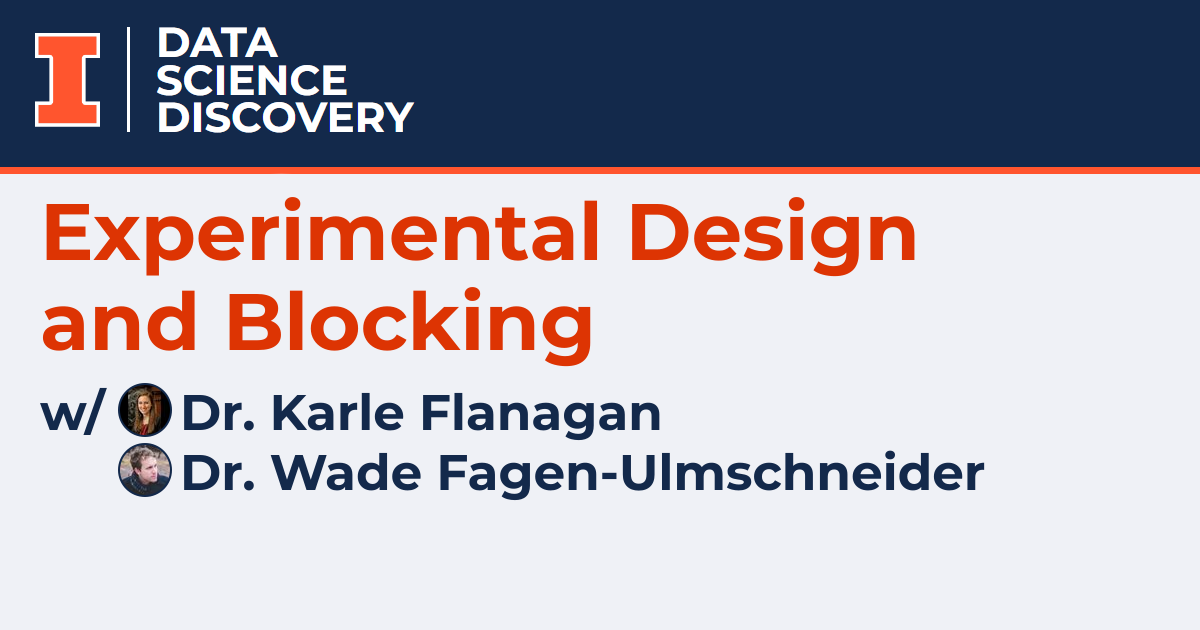
Experimental Design And Blocking Data Science Discovery Blocking first, then randomizing ensures that the treatment and control group are balanced with regard to the variables blocked on. if you think a variable could influence the response, you should block on that variable. a diagram showing an external design using no blocking (left side) vs. blocking (right side). The general idea behind cuped is that when you analyze your experiment, you can supplement the data that you collected during your experiment with additional data that was collected before the start of the experiment. in doing so, you can reduce the variance of the coefficient estimates in your experiment and effectively reduce the sample size. Create an exemplary data set for a completely randomized design with two treatments, four experimental units per treatment (replicates), and four observational units per experimental unit. compute the noncentrality parameter of the f distribution with the appropriate degrees of freedom under the alternative hypothesis that the two treatments. In the statistical theory of the design of experiments, blocking is the arranging of experimental units in groups (blocks) that are similar to one another. typically, a blocking factor is a source of variability that is not of primary interest to the experimenter. [3][4] no blocking (left) vs blocking (right) experimental design.

Introduction To Blocking In Experimental Design Youtube Create an exemplary data set for a completely randomized design with two treatments, four experimental units per treatment (replicates), and four observational units per experimental unit. compute the noncentrality parameter of the f distribution with the appropriate degrees of freedom under the alternative hypothesis that the two treatments. In the statistical theory of the design of experiments, blocking is the arranging of experimental units in groups (blocks) that are similar to one another. typically, a blocking factor is a source of variability that is not of primary interest to the experimenter. [3][4] no blocking (left) vs blocking (right) experimental design.
Comments are closed.