Explainable Ai Xai Engati
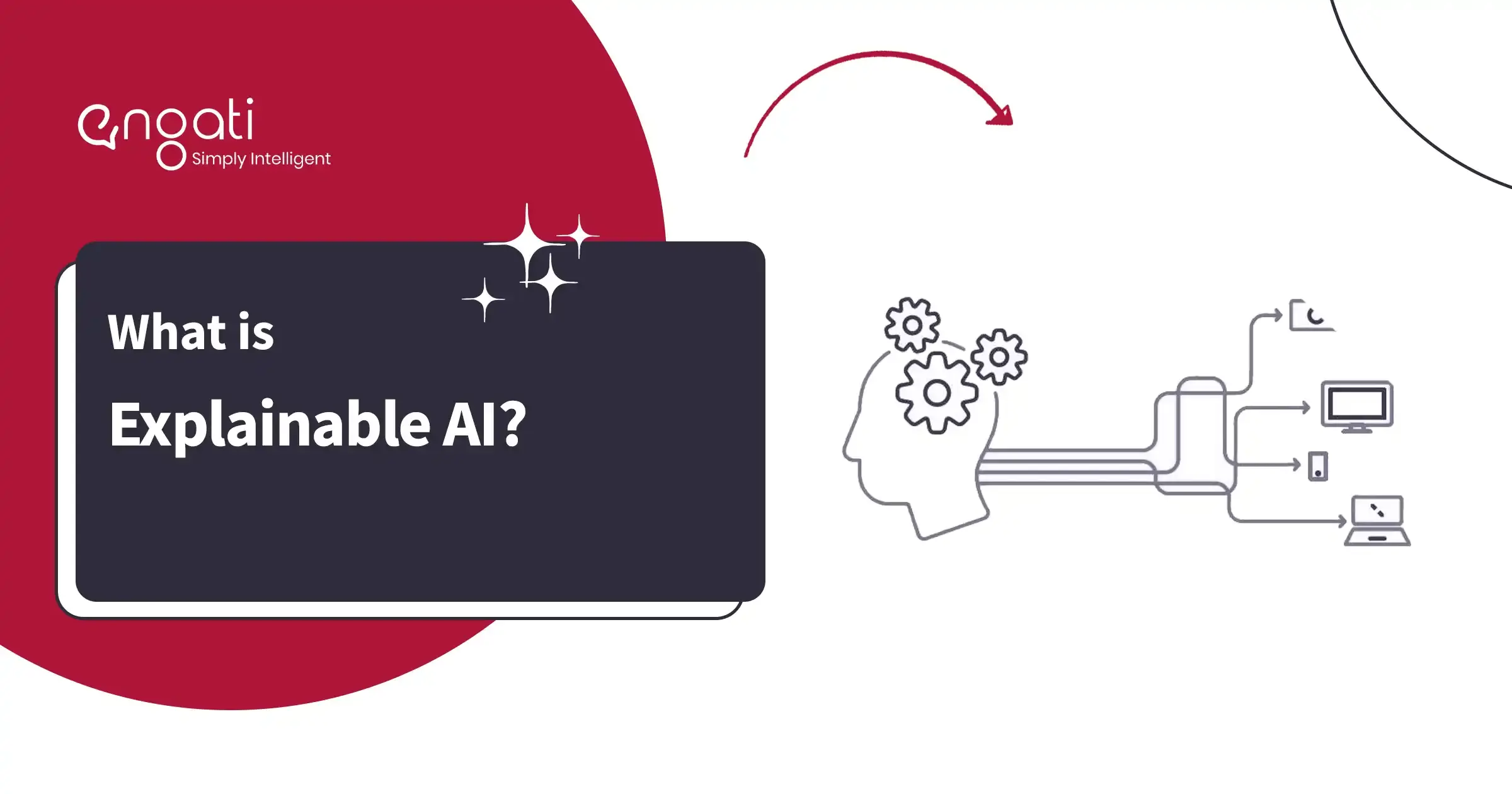
Explainable Ai Xai Engati Explainable ai also known as xai, is the practice using a set of techniques and approaches to design ai models in such a manner that the model’s output and decision making process is more transparent, interpretable and understandable to humans. What is explainable ai? explainable artificial intelligence, or xai, is a set of processes and methods that allow us to comprehend and trust the results and output created by machine learning algorithms. explainable ai is used to describe an ai model, its expected impact, and potential biases. it helps characterize model accuracy, fairness.
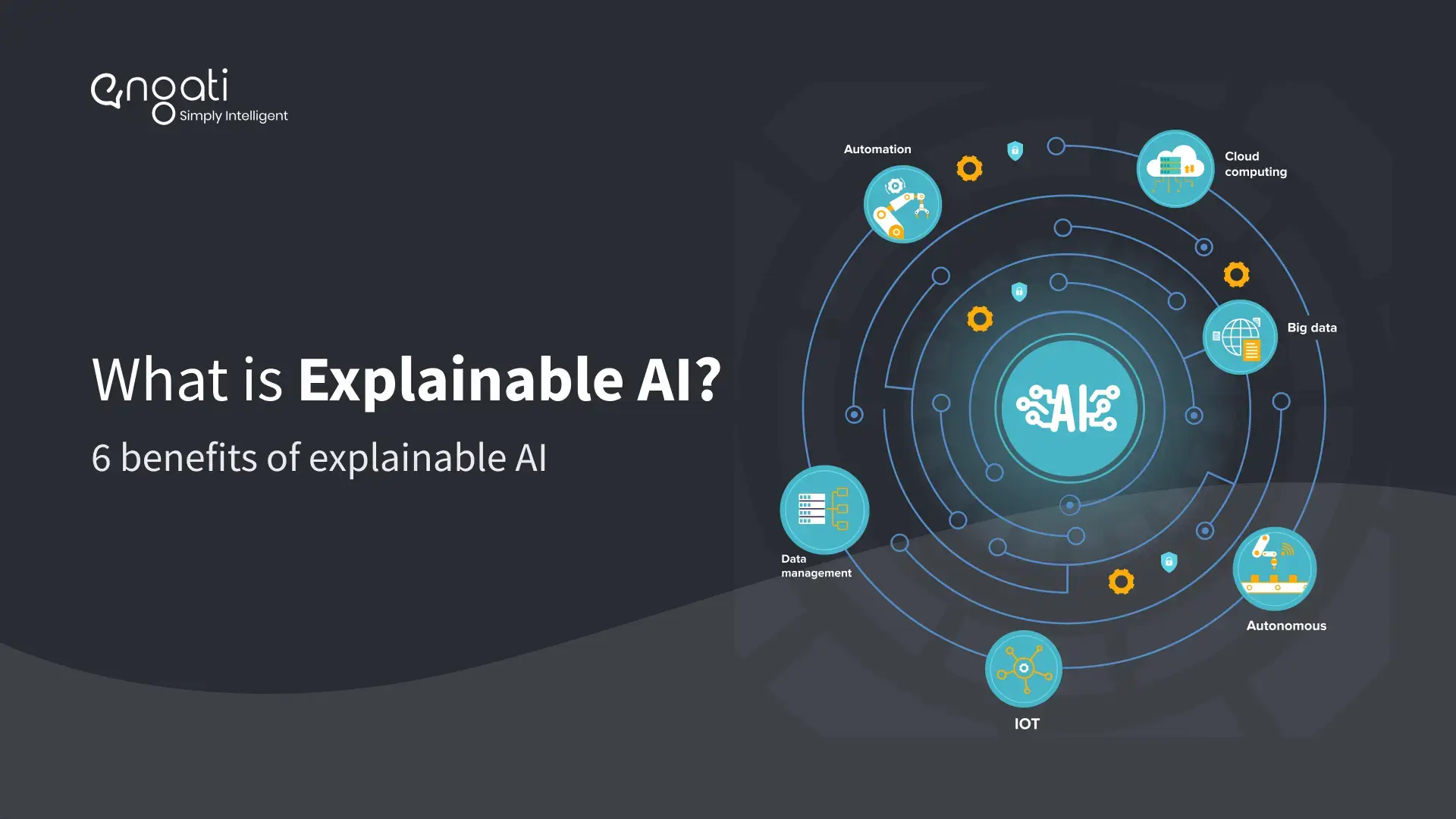
What Is Explainable Ai 6 Benefits Of Explainable Ai Engati 1.1. the black box issue and solution. the ai community is more concerned about the black box issue following the establishment of rules for trustworthy ais that are safe to use. explainable artificial intelligence (xai) techniques are aimed at producing ml models with a good interpretability accuracy tradeoff via: (i) building white gray box ml models which are interpretable by design (at. Progress in explainable artificial intelligence (xai) has been of great interest, particularly in light of the increasing use of ai systems in various fields, such as transparency requirements. Recent years have seen a rapid growth in the explainable ai (xai) literature. given the great variety of explanation models and the diversity of interpretations they can generate (sundararajan & najmi, 2020), decision makers are increasingly demanding that xai methods be designed for a single application. Here are some explainable ai principles that can contribute to building trust: transparency. ensuring stakeholders understand the models’ decision making process. fairness. ensuring that the models’ decisions are fair for everyone, including people in protected groups (race, religion, gender, disability, ethnicity).
Comments are closed.