Exploring Vector Databases The Next Step In Efficiently Handling High
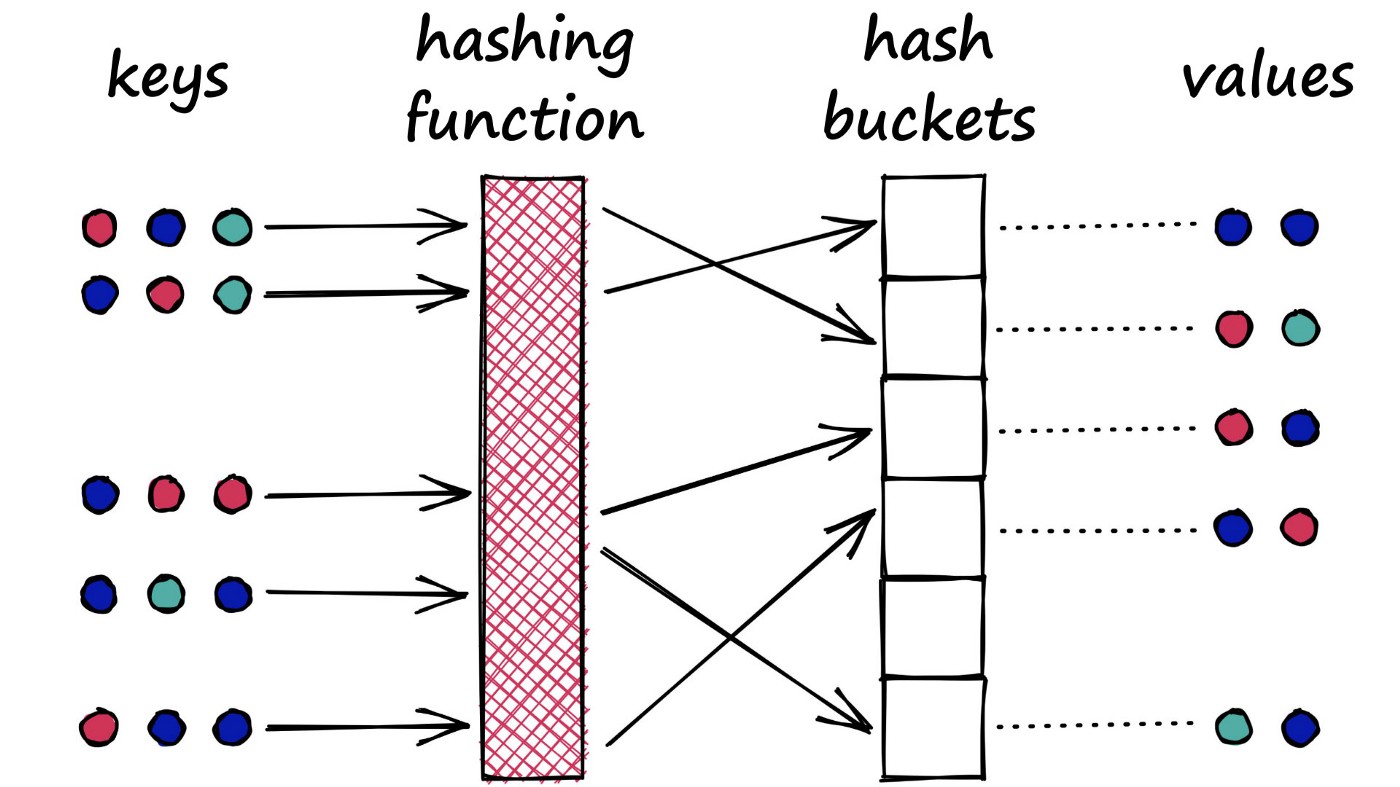
Exploring Vector Databases The Next Step In Efficiently Handling High Pinecone is a cloud native vector database that is built for handling high dimensional vectors. it is designed to be fast, scalable, and easy to use. pinecone can handle millions or even billions. Vector databases, as the name suggests, are databases optimized for handling vector data efficiently. vector data consists of elements like vectors, matrices, arrays, or tensors.

Exploring Vector Databases The Next Step In Efficiently Handling High Vector databases are revolutionizing data management by efficiently handling high dimensional data. they offer flexibility, excel in similarity searches, scale for big data, and enhance accuracy. The benefits of vector databases. let’s delve into the key advantages vector databases offer over nosql databases for high dimensional unstructured data: 1. efficient storage: vector databases are optimized for storing high dimensional data. nosql databases, while versatile, may not be as efficient when dealing with data that doesn’t fit. 1. vector indexing: vector databases use advanced indexing techniques like lsh (locality sensitive hashing) or hnsw (hierarchical navigable small world) to allow efficient searches across high. The purpose of vector databases in machine learning applications is to store and retrieve vector representations of various data elements, such as words, images, documents, or user preferences. unlike conventional databases that organize data in tabulated lists, vector databases store data and organize it using high dimensional vectors.
Exploring The Need For Vector Databases Its Relation With Llms 1. vector indexing: vector databases use advanced indexing techniques like lsh (locality sensitive hashing) or hnsw (hierarchical navigable small world) to allow efficient searches across high. The purpose of vector databases in machine learning applications is to store and retrieve vector representations of various data elements, such as words, images, documents, or user preferences. unlike conventional databases that organize data in tabulated lists, vector databases store data and organize it using high dimensional vectors. A vector database is a specialized type of database designed to store and manage high dimensional vectors. these vectors are numerical representations that capture the essence of data points in a multi dimensional space, including size and direction. developers can think of vectors as just an array of numbers. Vector databases represent a significant leap in data management technology, particularly in their application to ai and machine learning. by efficiently handling high dimensional vectors, these databases have become essential in the operation and development of advanced ai systems, including llms, generative ai, and deep learning.
Comments are closed.