Fine Tuning Your Draft

Fine Tuning Your Draft Youtube The first method of fine tuning rankings is a bit tedious. it involves manually editing the projections for individual players. but if you’re super optimistic about someone, adjusting their projected points will move that player up in your rankings and guarantee you an opportunity to draft him before anyone else has a chance to. Direct reward fine tuning (draft) is a simple and effective method for fine tuning diffusion models to maximize differentiable reward functions, as presented in directly fine tuning diffusion models on differentiable rewards. this post explains draft methods for diffusion models to better align with diverse and complex prompts.
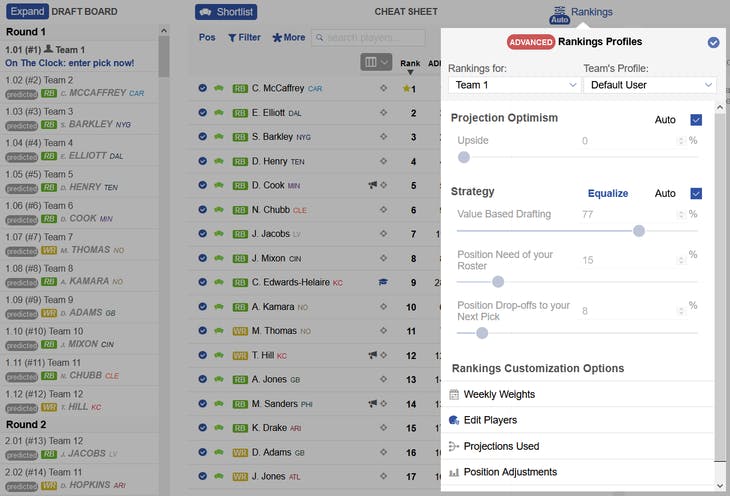
Draft Dominator Fine Tuning Rankings And Exploiting Strength Of Fine tuning rankings: the first method of fine tuning rankings is a bit tedious. it involves manually editing the projections for individual players. but if you’re super optimistic about someone, adjusting their projected points will move that player up in your rankings and guarantee you an opportunity to draft him before anyone else can. Adjusting the draft dominator's powerful ranking system for your own preferences. @fbgapps simon, lead apps engineer, takes you through it.get the draft domi. Don’t say what you don’t mean.” choose your words carefully and make them count. don’t belabor these details in your first draft; just get the writing on the page. but when you begin fine tuning during your revisions, take the time to cut down clunky writing, choose stronger nouns and verbs, and eliminate repetition. The above image demonstrates draft using human preference reward models. furthermore, the authors introduce enhancements to the draft method to enhance its efficiency and performance. first, they propose draft k, a variant that limits backpropagation to only the last k steps of sampling when computing the gradient for fine tuning.
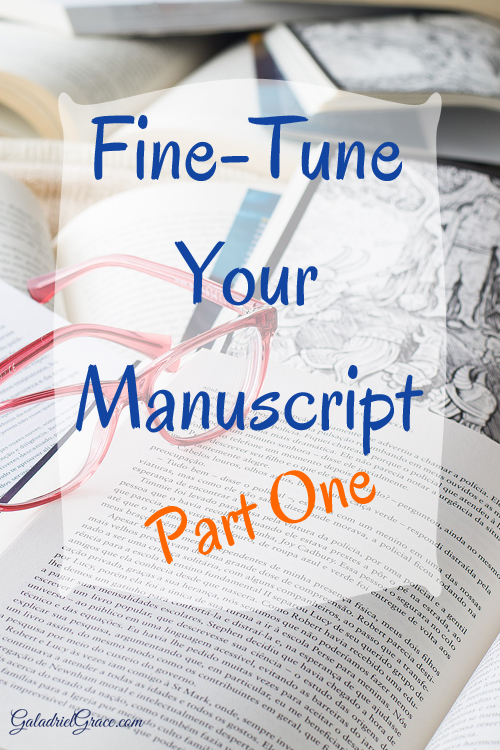
Fine Tuning Your Manuscript вђ Part One Galadriel Grace Don’t say what you don’t mean.” choose your words carefully and make them count. don’t belabor these details in your first draft; just get the writing on the page. but when you begin fine tuning during your revisions, take the time to cut down clunky writing, choose stronger nouns and verbs, and eliminate repetition. The above image demonstrates draft using human preference reward models. furthermore, the authors introduce enhancements to the draft method to enhance its efficiency and performance. first, they propose draft k, a variant that limits backpropagation to only the last k steps of sampling when computing the gradient for fine tuning. We present direct reward fine tuning (draft), a simple and effective method for fine tuning diffusion models to maximize differentiable reward functions, such as scores from human preference models. we first show that it is possible to backpropagate the reward function gradient through the full sampling procedure, and that doing so achieves strong performance on a variety of rewards. According to openai fine tuning documentation, there are a number of models that can be fine tuned. these include: gpt 4o mini 2024 07 18: with a 65k training context window. gpt 3.5 turbo 1106: with a 16k context window. gpt 3.5 turbo 0613: with a 4k context window.

Chapter 5 Nonfiction Narration Ppt Download We present direct reward fine tuning (draft), a simple and effective method for fine tuning diffusion models to maximize differentiable reward functions, such as scores from human preference models. we first show that it is possible to backpropagate the reward function gradient through the full sampling procedure, and that doing so achieves strong performance on a variety of rewards. According to openai fine tuning documentation, there are a number of models that can be fine tuned. these include: gpt 4o mini 2024 07 18: with a 65k training context window. gpt 3.5 turbo 1106: with a 16k context window. gpt 3.5 turbo 0613: with a 4k context window.
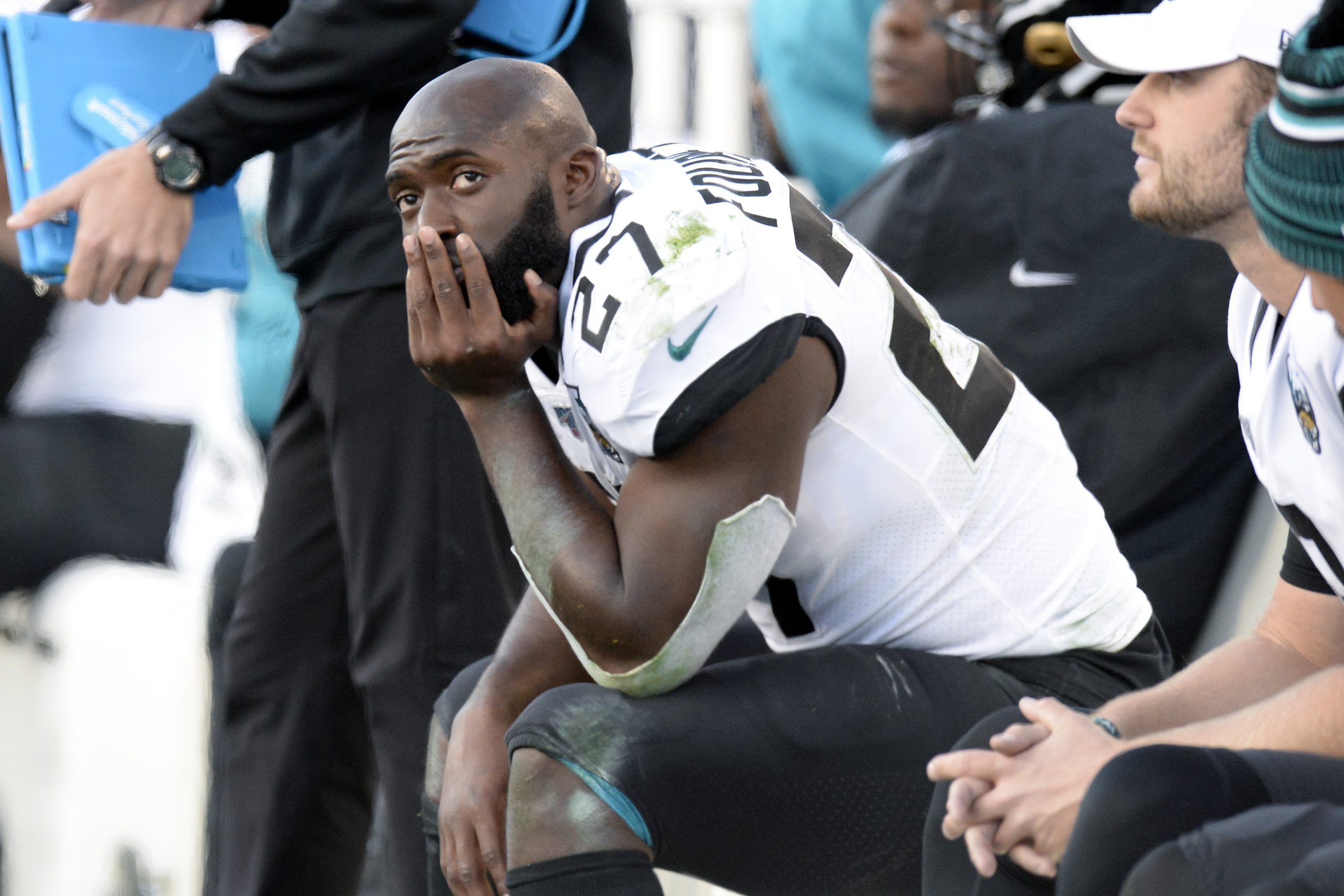
Fantasy Football Intelligence Fine Tuning Your Draft The Pulse Of Nh
Comments are closed.