Hmm Hidden Markov Model
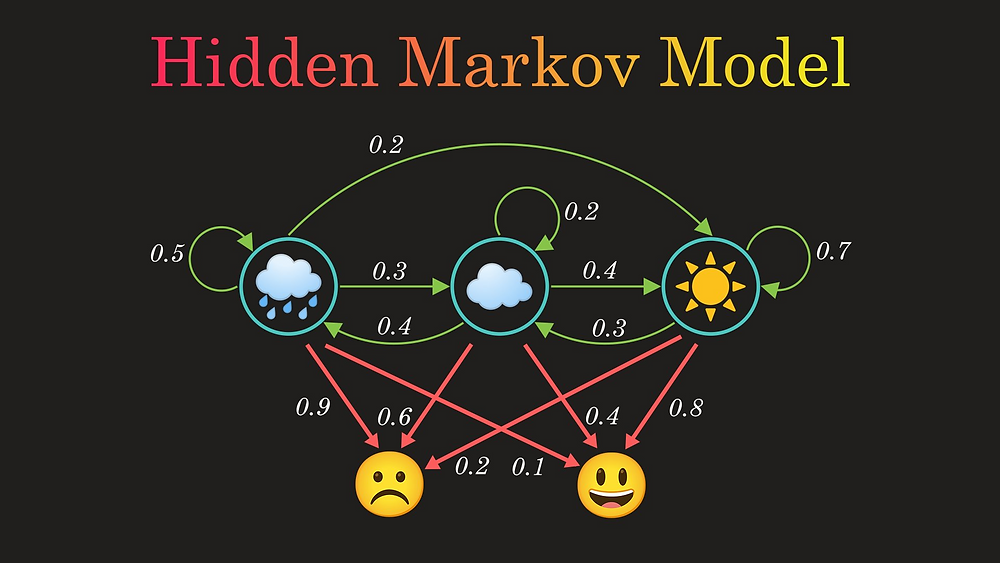
Introduction To Hidden Markov Model Hmm And Its Application In Stock A hidden markov model (hmm) is a markov model in which the observations are dependent on a latent (or "hidden") markov process (referred to as ). an hmm requires that there be an observable process y {\displaystyle y} whose outcomes depend on the outcomes of x {\displaystyle x} in a known way. In next section i will explain these hmm parts in details. hidden states and observation symbols. hmm has two parts: hidden and observed. the hidden part consist of hidden states which are not directly observed, their presence is observed by observation symbols that hidden states emits. example 1. you don’t know in what mood your girlfriend.
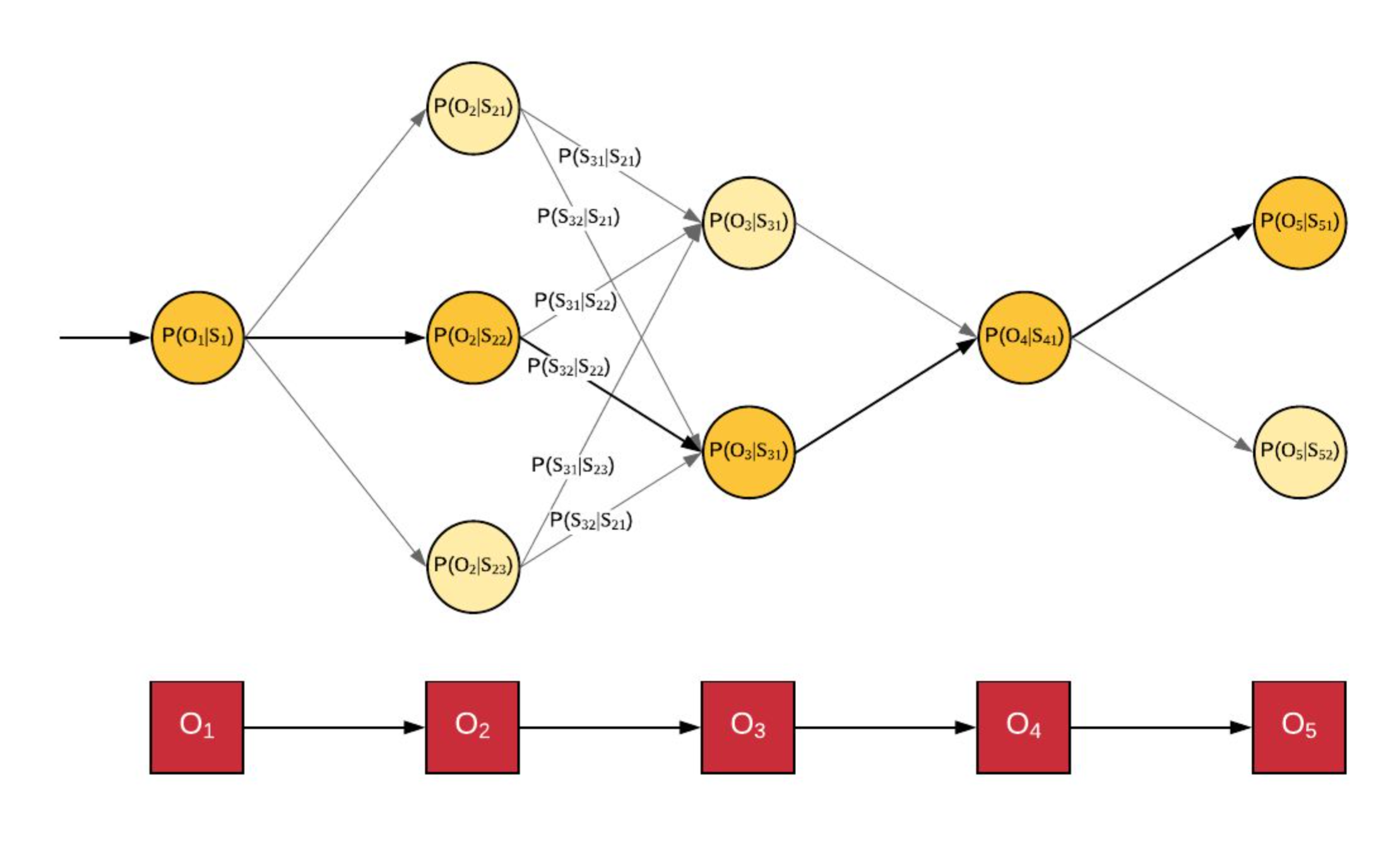
Hidden Markov Models вђ Tanmay Binaykiya Hidden markov models, known as hmm for short, are statistical models that work as a sequence of labeling problems. these are the types of problems that describe the evolution of observable events, which themselves, are dependent on internal factors that can’t be directly observed — they are hidden [3]. A hidden markov model is a tool for representing prob ability distributions over sequences of observations [1]. in this model, an observation x t at time tis produced by a stochastic process, but the state z tof this process cannot be directly observed, i.e. it is hidden [2]. this hidden process is assumed to satisfy the markov property, where. Hidden markov models. in this section, we discuss the hidden markov model or hmm, which is a state space model in which the hidden states are discrete, so x t ∈ {1, …, n s}. the observations may be discrete, y t ∈ {1, …, n y}, or continuous, y t ∈ r s n, or some combination, as we illustrate below. Hidden markov models (hmms) are a formal foundation for making probabilistic models of linear sequence 'labeling' problems 1, 2. they provide a conceptual toolkit for building complex models just.

Hidden Markov Model Hmm In Nlp Complete Implementation In Python Hidden markov models. in this section, we discuss the hidden markov model or hmm, which is a state space model in which the hidden states are discrete, so x t ∈ {1, …, n s}. the observations may be discrete, y t ∈ {1, …, n y}, or continuous, y t ∈ r s n, or some combination, as we illustrate below. Hidden markov models (hmms) are a formal foundation for making probabilistic models of linear sequence 'labeling' problems 1, 2. they provide a conceptual toolkit for building complex models just. A hidden markov model (hmm) can be used to explore this scenario. we don't get to observe the actual sequence of states (the weather on each day). rather, we can only observe some outcome generated by each state (how many ice creams were eaten that day). ormallyf, an hmm is a markov model for which we have a series of observed outputs x= fx 1;x. A hidden markov model (hmm) is one in which you observe a sequence of emissions, but do not know the sequence of states the model went through to generate the emissions. analyses of hidden markov models seek to recover the sequence of states from the observed data. as an example, consider a markov model with two states and six possible emissions.

Ppt Hidden Markov Model An Introduction Powerpoint Presentation A hidden markov model (hmm) can be used to explore this scenario. we don't get to observe the actual sequence of states (the weather on each day). rather, we can only observe some outcome generated by each state (how many ice creams were eaten that day). ormallyf, an hmm is a markov model for which we have a series of observed outputs x= fx 1;x. A hidden markov model (hmm) is one in which you observe a sequence of emissions, but do not know the sequence of states the model went through to generate the emissions. analyses of hidden markov models seek to recover the sequence of states from the observed data. as an example, consider a markov model with two states and six possible emissions.
Comments are closed.