Inference Of Stochastic Dexter Barrows General Computational

Inference Of Stochastic Dexter Barrows General Computational Inference of stochastic biochemical system reaction rates dexter barrows general computational biology ismb 2020 posters. Dexter barrows. dbarrows. research scientist at ndi, and phd candidate in modelling and simulation of stochastic biochemical networks at toronto metropolitan university. prevent this user from interacting with your repositories and sending you notifications. learn more about.
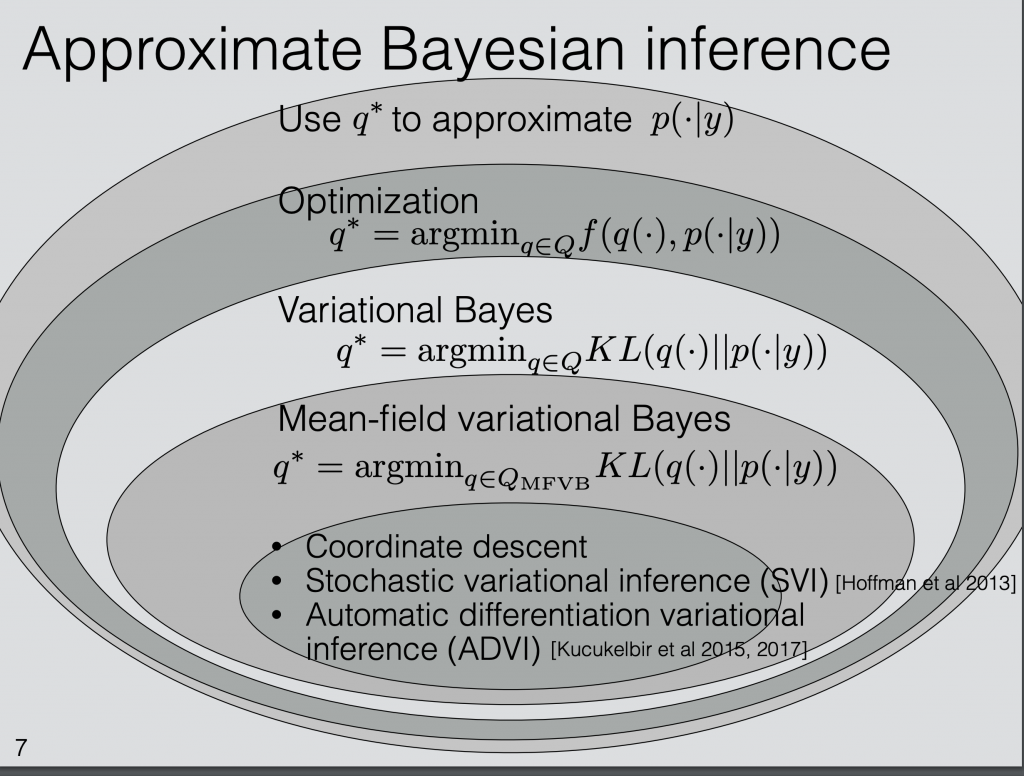
Stochastic Variational Inference вђ Czxttkl M.sc. thesis dexter barrows mcmaster university mathematics 6.4 runtimes for producing sirs forecasts. the box shows the middle 50th percent, the bold line is the median, and the dots are outliers. note that these are not \true" outliers, simply ones outside a threshold based. A comparative study of techniques for estimation and inference of nonlinear stochastic time series: authors: barrows, dexter: advisor: bolker, benjamin: department: mathematics and statistics: keywords: forecasting, time series, estimation, fitting: publication date: 2016: abstract: forecasting tools play an important role in public response to. The yuima project is an open source and collaborative effort aimed at developing the r package yuima for simulation and inference of stochastic differential equations. in the yuima package stochastic differential equations can be of very abstract type, multidimensional, driven by wiener process or fractional brownian motion with general hurst parameter, with or without jumps specified as lévy. In this work, we extend techniques designed to infer model parameters of partially observed markov processes to the problem of stochastic biochemical system reaction and diffusion rate inference, given partial and or sparse observation data. 25 these techniques are especially well suited to the problem of fitting models to observation data that may be noisy, such as those obtained from.
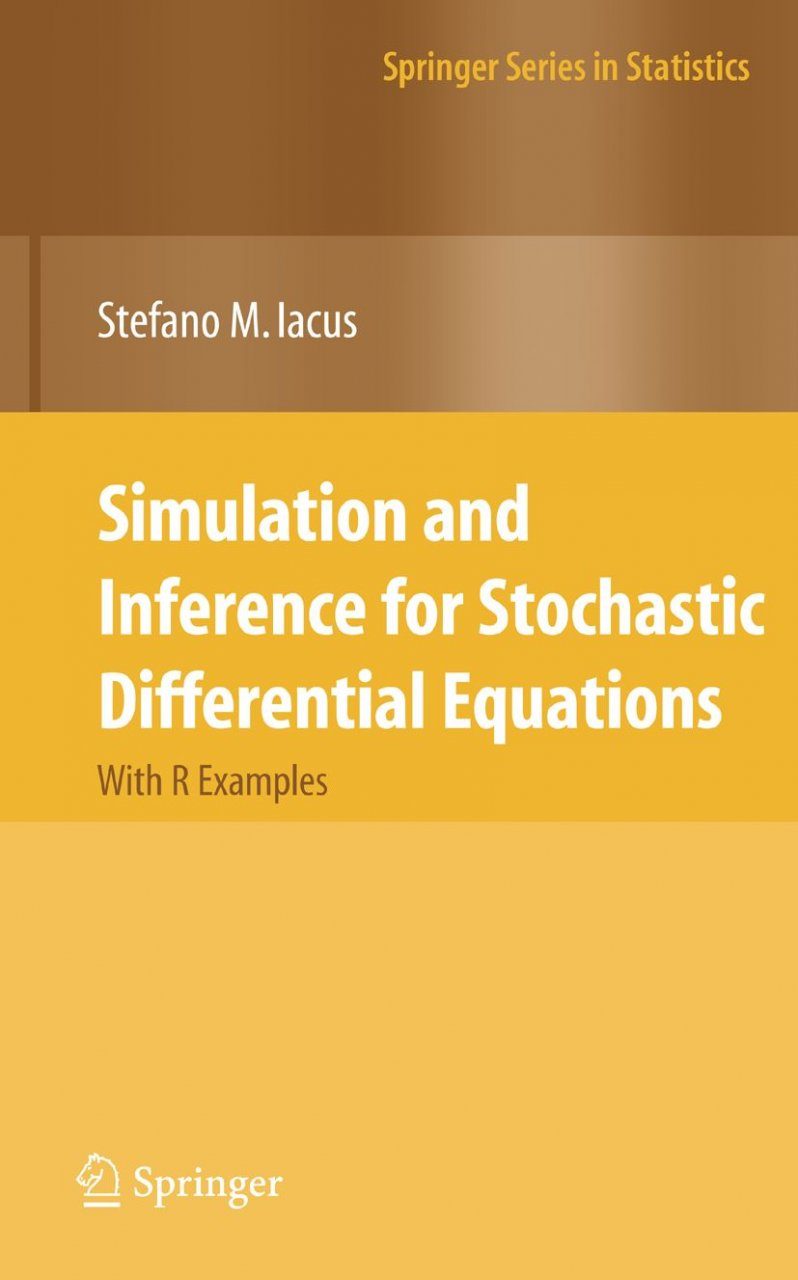
Simulation And Inference For Stochastic Differential Equations Nhbs The yuima project is an open source and collaborative effort aimed at developing the r package yuima for simulation and inference of stochastic differential equations. in the yuima package stochastic differential equations can be of very abstract type, multidimensional, driven by wiener process or fractional brownian motion with general hurst parameter, with or without jumps specified as lévy. In this work, we extend techniques designed to infer model parameters of partially observed markov processes to the problem of stochastic biochemical system reaction and diffusion rate inference, given partial and or sparse observation data. 25 these techniques are especially well suited to the problem of fitting models to observation data that may be noisy, such as those obtained from. The stochastic robust optimization framework is able to organically integrate the two stage stochastic programming approach with the aro method and leverage their strengths. a general form of the stochastic robust milp is given by (yue and you, 2016), (4) min x c t x e σ ∈ Π [c ¯ σ (x)] s. t. ax ≥ d x ∈ r n 1 × z n 2 {c ¯ σ (x. Hierarchical bayesian modeling is a general approach to handling model uncertainty whereby full stochastic dynamical models are represented as hierarchies of simpler, analytically tractable sub models [24], [25]. if these sub models are properly formulated, inference can be performed separately for each by exploiting their conditional independences, with the sub models aggregated afterwards to.
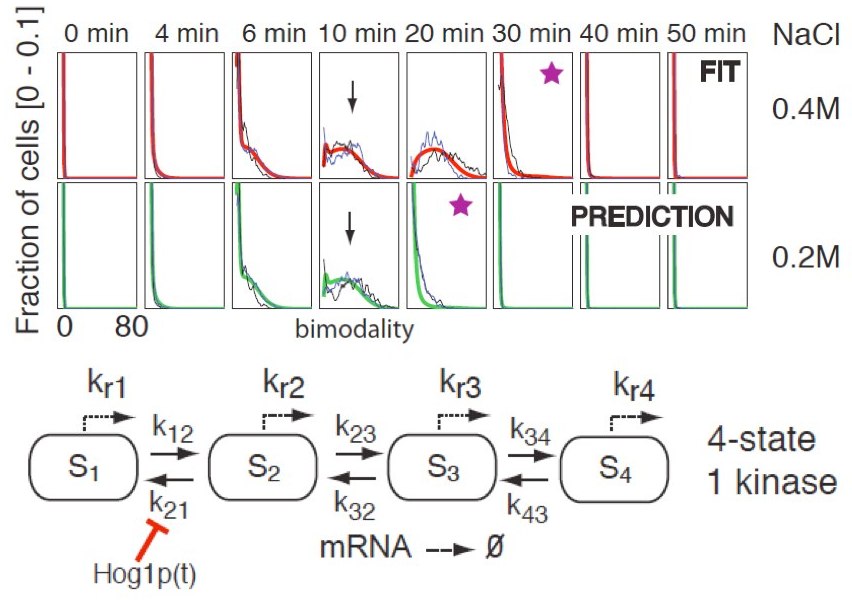
Model And Parameter Inference вђ Control Theory And Systems Biology The stochastic robust optimization framework is able to organically integrate the two stage stochastic programming approach with the aro method and leverage their strengths. a general form of the stochastic robust milp is given by (yue and you, 2016), (4) min x c t x e σ ∈ Π [c ¯ σ (x)] s. t. ax ≥ d x ∈ r n 1 × z n 2 {c ¯ σ (x. Hierarchical bayesian modeling is a general approach to handling model uncertainty whereby full stochastic dynamical models are represented as hierarchies of simpler, analytically tractable sub models [24], [25]. if these sub models are properly formulated, inference can be performed separately for each by exploiting their conditional independences, with the sub models aggregated afterwards to.
Comments are closed.