Lecture 14 Expectation Maximization Algorithms
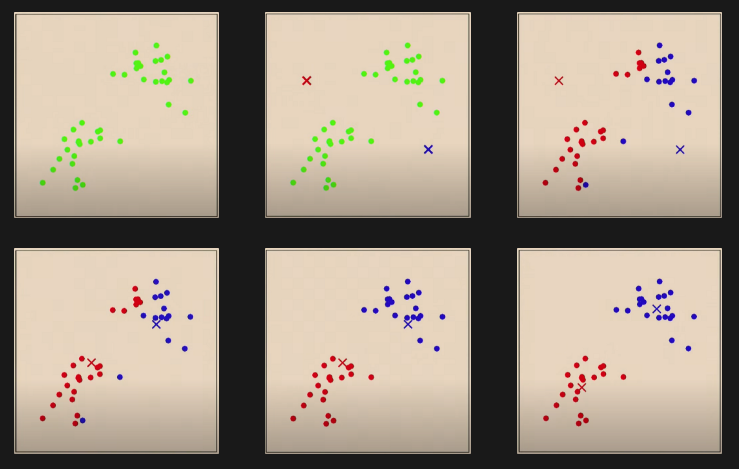
Lecture 14 Expectation Maximization Algorithms The expectation maximization algorithm enables parameter estimation in probabilistic models with incomplete data In summary, the expectation maximization algorithm alternates between the steps of Example 2C1 (Note: The values of P and F_2 given in the lecture book should be switched for this problem The solution here is in terms of variables, not numbers, and is correct)

Lecture 14 Expectation Maximization Algorithms Stanford Cs229 To save content items to your account, please confirm that you agree to abide by our usage policies If this is the first time you use this feature, you will be asked to authorise Cambridge Core to This series provides a vehicle for the publication of informal lecture note volumes in all areas of theoretical and experimental physics Aimed at graduate students and researchers, the notes present Fringe theories such as QAnon have increasingly become the norm among Americans as smart phones, algorithms and augmented reality impact our abilities to decipher between truth and fiction When you create an algorithm, you need to include precise, step-by-step instructions This means you will need to break down the task or problem into smaller steps We call this process
Comments are closed.