Pdf Multiple Alignment Using Hidden Markov Models Semantic Scholar
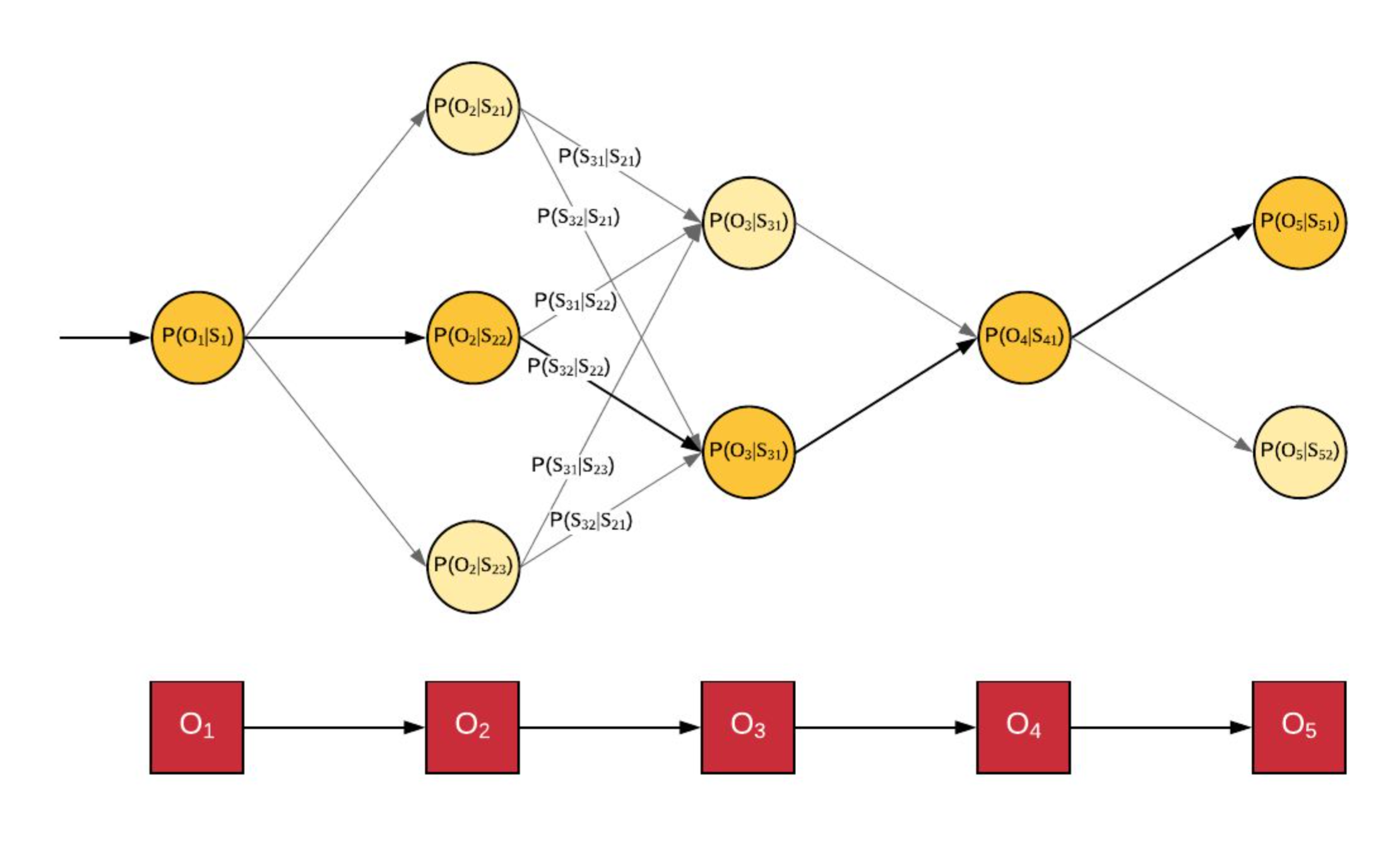
Hidden Markov Models вђ Tanmay Binaykiya A bayesian method for estimating the amino acid distributions in the states of a hidden markov model (hmm) for a protein family or the columns of a multiple alignment of that family is introduced, which can improve the quality of hmms produced from small training sets. expand. 220. pdf. 1 excerpt. Mals improves alignment quality by using pairwise alignment hidden markov models with multiple match states that describe local structural information without exploiting explicit structure predictions, and correlates well with reference dependent evaluation that compares sequence based alignments to structure based references. we have developed mummals, a program to construct multiple protein.
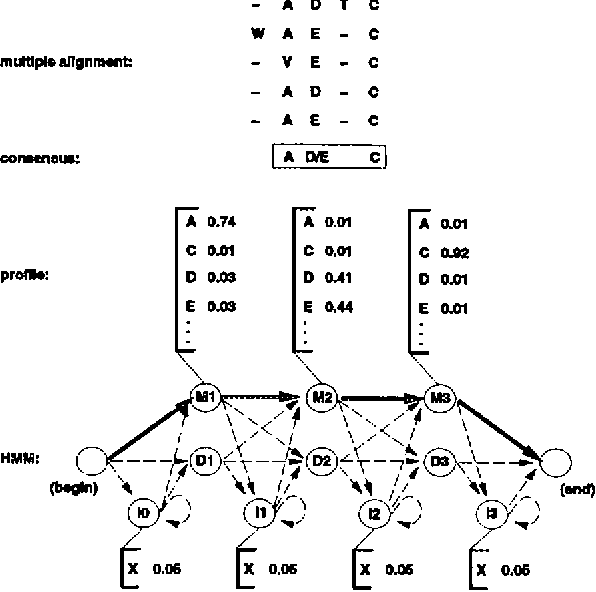
Pdf Multiple Alignment Using Hidden Markov Models Semantic Scholar Hidden markov models. published in methods in molecular biology 28 january 2005. biology, computer science. hidden markov models (hmms) are a highly effective means of modeling a family of unaligned sequences or a common motif within a set of unaligned sequences. the trained hmm can then be used for discrimination or multiple alignment. Contents. a simulated annealing method is described for training hidden markov models and producing multiple sequence alignments from initially unaligned protein or dna sequences. simulated annealing in turn uses a dynamic programming algorithm for correctly sampling suboptimal multiple alignments according to their probability and a boltzmann. Background during procedures for conducting multiple sequence alignment, that is so essential to use the substitution score of pairwise alignment. to compute adaptive scores for alignment, researchers usually use hidden markov model or probabilistic consistency methods such as partition function. recent studies show that optimizing the parameters for hidden markov model, as well as integrating. Figure 1 diagrams the structure of a hidden markov model for modeling primary sequence consensus infor mation derived from multiple sequence alignments, as introduced by krogh et al. (1994). one match state is assigned to each consensus column of the multiple alignment. insert states insert extra symbols relative to the consensus match states.

Hidden Markov Model Background during procedures for conducting multiple sequence alignment, that is so essential to use the substitution score of pairwise alignment. to compute adaptive scores for alignment, researchers usually use hidden markov model or probabilistic consistency methods such as partition function. recent studies show that optimizing the parameters for hidden markov model, as well as integrating. Figure 1 diagrams the structure of a hidden markov model for modeling primary sequence consensus infor mation derived from multiple sequence alignments, as introduced by krogh et al. (1994). one match state is assigned to each consensus column of the multiple alignment. insert states insert extra symbols relative to the consensus match states. 1 f32 gm16932 01 gm nigms nih hhs united states. a simulated annealing method is described for training hidden markov models and producing multiple sequence alignments from initially unaligned protein or dna sequences. simulated annealing in turn uses a dynamic programming algorithm for correctly sampling suboptimal multiple alignments. Therefore, many heuristics have been proposed to compute nearly optimal alignments, such as progressive alignment (feng and doolittle, 1987), iterative alignment (barton and sternberg, 1987; berger and munson, 1991; corpet, 1988; subbiah and harrison, 1989) and alignment based on profile hidden markov models (krogh et al., 1994; rabiner, 1989.
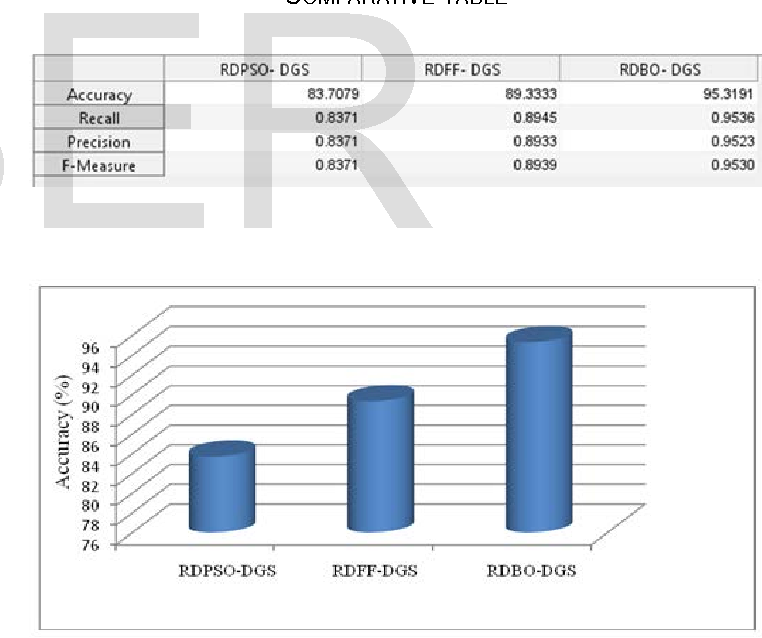
Table 1 From A Comparative Study Of Hidden Markov Models Learned By 1 f32 gm16932 01 gm nigms nih hhs united states. a simulated annealing method is described for training hidden markov models and producing multiple sequence alignments from initially unaligned protein or dna sequences. simulated annealing in turn uses a dynamic programming algorithm for correctly sampling suboptimal multiple alignments. Therefore, many heuristics have been proposed to compute nearly optimal alignments, such as progressive alignment (feng and doolittle, 1987), iterative alignment (barton and sternberg, 1987; berger and munson, 1991; corpet, 1988; subbiah and harrison, 1989) and alignment based on profile hidden markov models (krogh et al., 1994; rabiner, 1989.
Comments are closed.