Privacy Preserving Ai Private Ai вђ The Rise Of Federated Learning
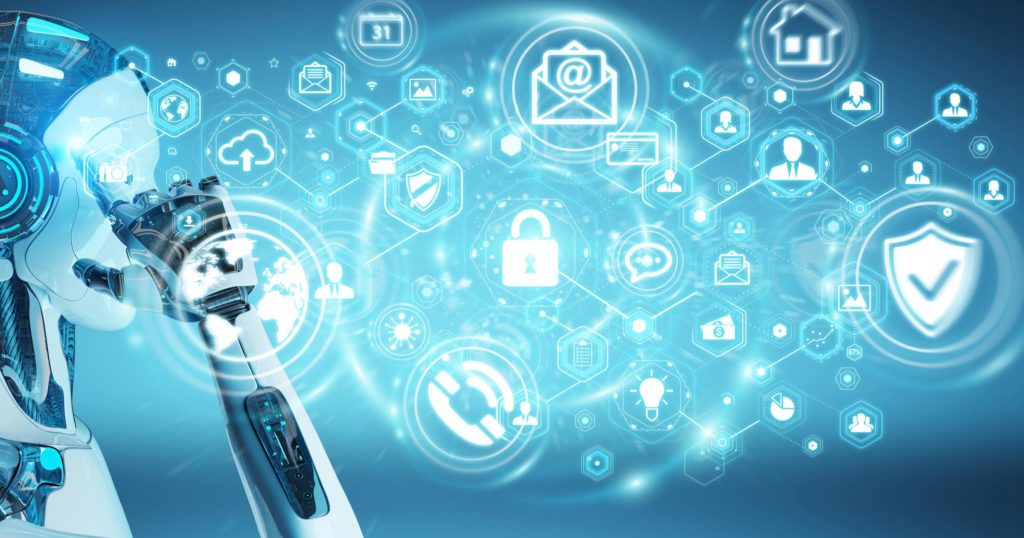
Privacy Preserving Ai Private Ai вђ The Rise Of Federated Bhushan garware@persistent . machine learning (13) artificial intelligence (11) ai (4) cloud based service (1) federated learning (1) federated learning is a technique to train machine learning models on data to which you do not have access. instead of collecting all data within a central cloud and building a model, we will bring the model. Federated learning for privacy preserving ai. authors: yong cheng, yang liu, tianjian chen, qiang yang authors info & claims. communications of the acm, volume 63, issue 12. pages 33 36.
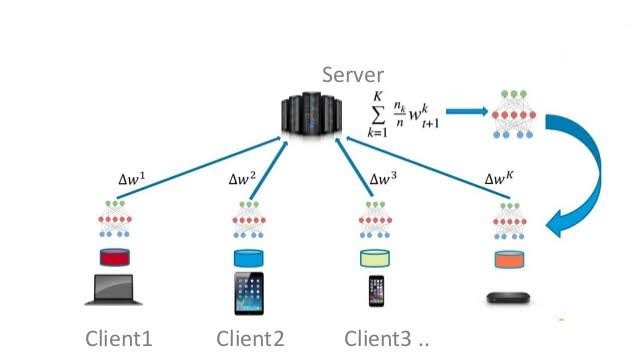
Privacy Preserving Ai Private Ai вђ The Rise Of Federated In the past decades, artificial intelligence (ai) has achieved unprecedented success, where statistical models become the central entity in ai. however, the centralized training and inference paradigm for building and using these models is facing more and more privacy and legal challenges. to bridge the gap between data privacy and the need for data fusion, an emerging ai paradigm federated. Privacy preserving ml techniques using tees (sec. 2.3), as well as core ideas behind layer wise dnn training for fl (sec. 2.4). 2.1 trusted execution environments (tee). Federated learning has emerged as a privacy preserving machine learning approach where multiple parties can train a single model without sharing their raw training data. federated learning typically requires the utilization of multi party computation techniques to provide strong privacy guarantees by ensuring that an untrusted or curious aggregator cannot obtain isolated replies from parties. Federated learning is a machine learning paradigm that emerges as a solution to the privacy preservation demands in artificial intelligence. as machine learning, federated learning is threatened by adversarial attacks against the.

Federated Learning A Key Technology For Privacy Preserving Ai By Federated learning has emerged as a privacy preserving machine learning approach where multiple parties can train a single model without sharing their raw training data. federated learning typically requires the utilization of multi party computation techniques to provide strong privacy guarantees by ensuring that an untrusted or curious aggregator cannot obtain isolated replies from parties. Federated learning is a machine learning paradigm that emerges as a solution to the privacy preservation demands in artificial intelligence. as machine learning, federated learning is threatened by adversarial attacks against the. Federated learning (fl), emerging as a distributed machine learning, is a popular paradigm that allows multiple users to collaboratively train an intermediate model by exchanging local models without the training data leaving each user’s domain. however, fl still suffer from privacy risk such as leaking private information from users’ uploaded local models. to address the privacy concern. 2022. tldr. this work proposes an efficient and privacy preserving federated learning solution that improves performance and security by offloading the aggregation procedure into the hardware data plane, like fpga based smartnics and also combines it with differential privacy techniques. expand.

Federated Learning Privacy Preserving Ai By Nunzio Logallo Federated learning (fl), emerging as a distributed machine learning, is a popular paradigm that allows multiple users to collaboratively train an intermediate model by exchanging local models without the training data leaving each user’s domain. however, fl still suffer from privacy risk such as leaking private information from users’ uploaded local models. to address the privacy concern. 2022. tldr. this work proposes an efficient and privacy preserving federated learning solution that improves performance and security by offloading the aggregation procedure into the hardware data plane, like fpga based smartnics and also combines it with differential privacy techniques. expand.
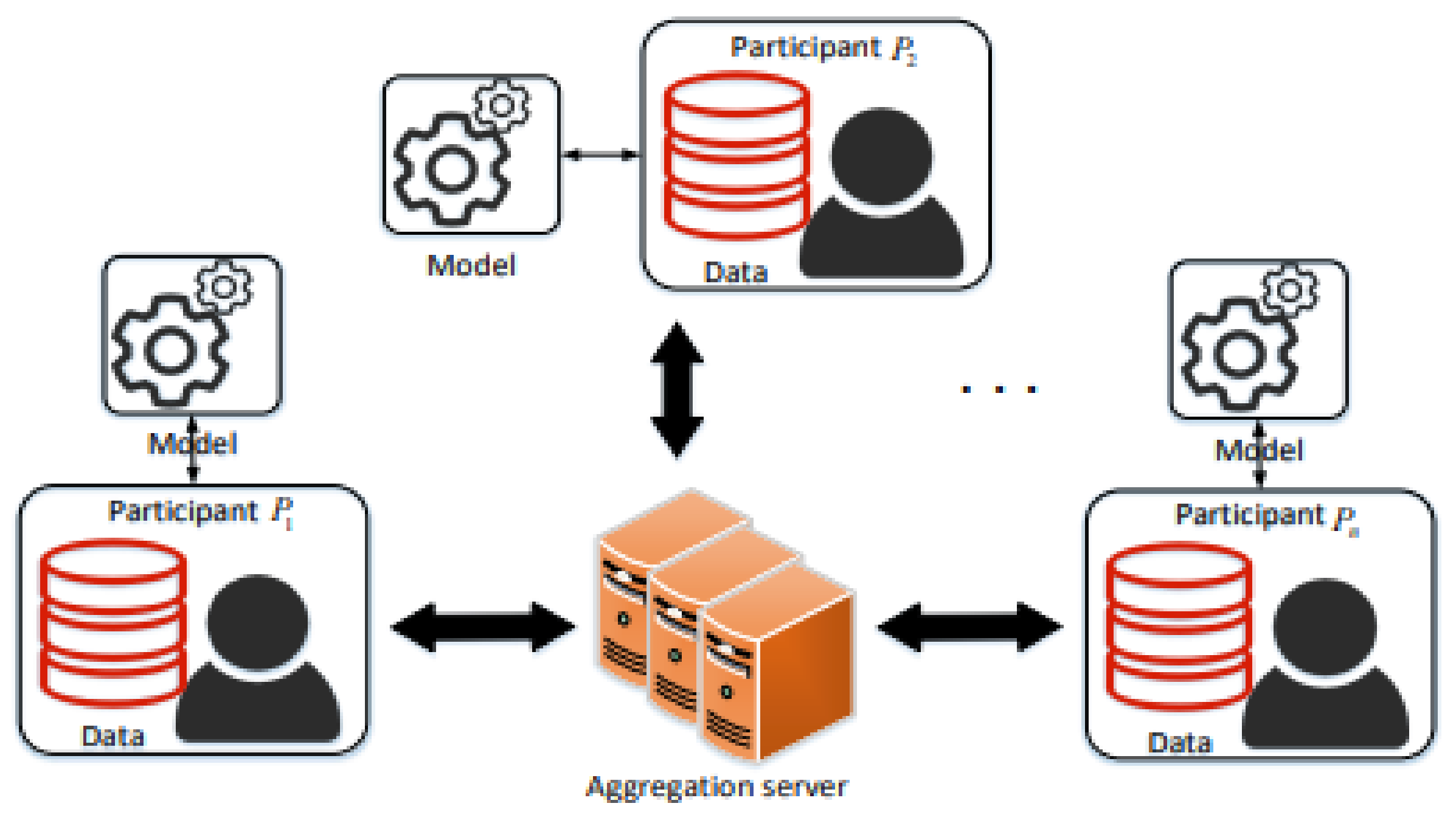
Privacy Preserving And Explainable Ai Encyclopedia Mdpi
Comments are closed.