Privacy Preserving Machine Learning Ppml Duality Technologies
Privacy Preserving In Machine Learning Ppml Analytics Vidhya The answer to the privacy ai conflict is privacy preserving machine learning (ppml) – a step by step process to allow ml models to be trained without revealing or decrypting the data inputs. the goal of ppml is to preserve the accuracy of the model without compromising on the quality of the model or on the quality of the underlying data. Machine learning (ml) is increasingly being adopted in a wide variety of application domains. usually, a well performing ml model relies on a large volume of training data and high powered computational resources. such a need for and the use of huge volumes of data raise serious privacy concerns because of the potential risks of leakage of highly privacy sensitive information; further, the.
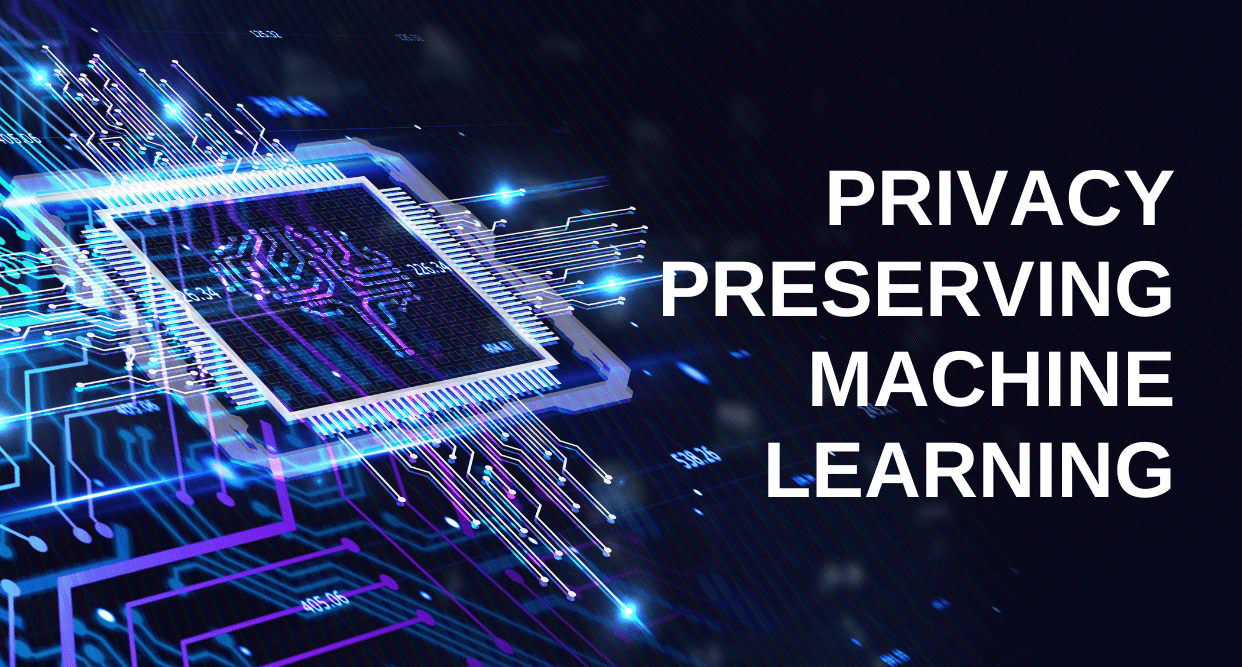
Privacy Preserving Machine Learning Ppml Duality Technologies Here, we proposed a secure and privacy preserving machine learning method (ppml omics) by designing a decentralized differential private federated learning algorithm. we applied ppml omics to analyze data from three sequencing technologies and addressed the privacy concern in three major tasks of omic data under three representative deep. Ppml solutions either claim the support of privacy preserving model creation or focus on privacy preserving model serving . as demonstrated in section 2.1, the computation tasks at the model. This one day workshop focuses on privacy preserving techniques for machine learning and disclosure in large scale data analysis, both in the distributed and centralized settings, and on scenarios that highlight the importance and need for these techniques (e.g., via privacy attacks). there is growing interest from the machine learning (ml. Scope. this one day workshop focuses on privacy preserving techniques for training, inference, and disclosure in large scale data analysis, both in the distributed and centralized settings. we have observed increasing interest of the machine learning (ml) community in leveraging cryptographic techniques such as multi party computation (mpc) and.
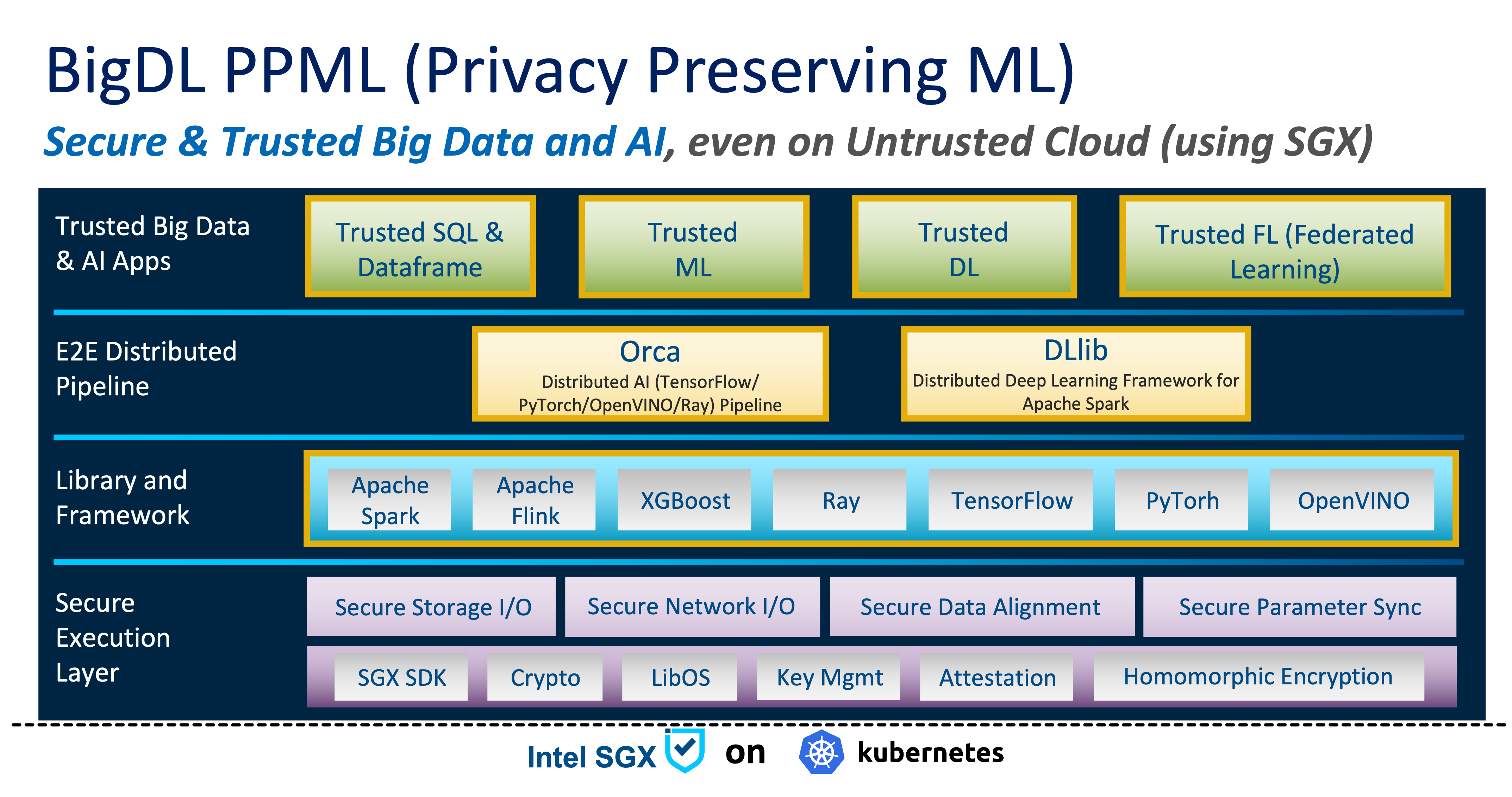
Bigdl Privacy Preserving Machine Learning Ppml вђ Confidential This one day workshop focuses on privacy preserving techniques for machine learning and disclosure in large scale data analysis, both in the distributed and centralized settings, and on scenarios that highlight the importance and need for these techniques (e.g., via privacy attacks). there is growing interest from the machine learning (ml. Scope. this one day workshop focuses on privacy preserving techniques for training, inference, and disclosure in large scale data analysis, both in the distributed and centralized settings. we have observed increasing interest of the machine learning (ml) community in leveraging cryptographic techniques such as multi party computation (mpc) and. This paper systematically review and summarize existing privacy preserving approaches and proposes a phase, guarantee, and utility (pgu) triad based model to understand and guide the evaluation of various ppml solutions by decomposing their privacy preserving functionalities. machine learning (ml) is increasingly being adopted in a wide variety of application domains. usually, a well. In this paper, we systematically review and summarize existing privacy preserving approaches and propose a phase, guarantee, and utility (pgu) triad based model to understand and guide the evaluation of various ppml solutions by decomposing their privacy preserving functionalities. we discuss the unique characteristics and challenges of ppml.
Comments are closed.