What Is U Net
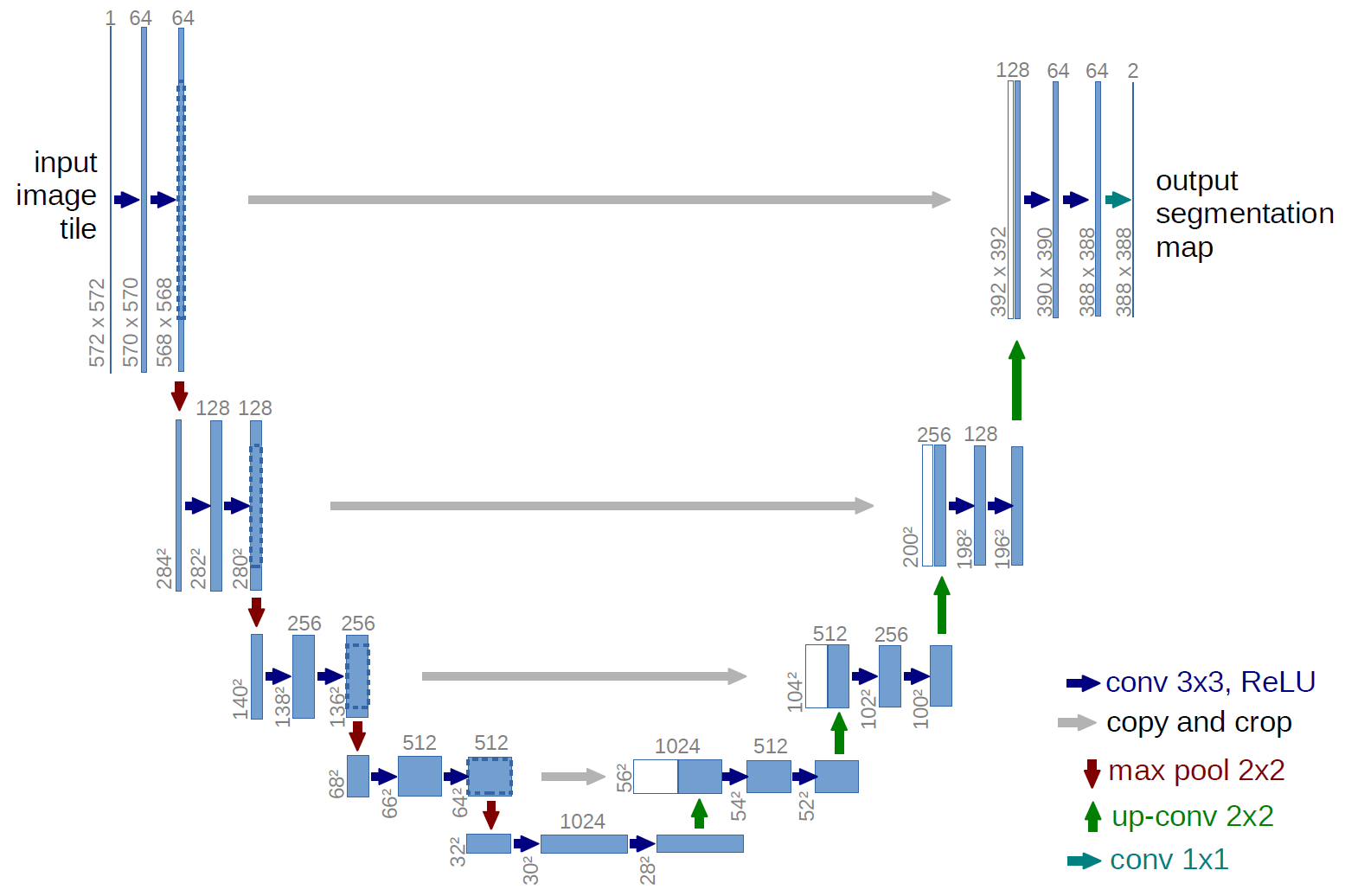
U Net Convolutional Networks For Biomedical Image Segmentation U net is a convolutional neural network that was developed for image segmentation. [1] the network is based on a fully convolutional neural network [ 2 ] whose architecture was modified and extended to work with fewer training images and to yield more precise segmentation . U net is a widely used deep learning architecture that was first introduced in the “u net: convolutional networks for biomedical image segmentation” paper. the primary purpose of this architecture was to address the challenge of limited annotated data in the medical field. this network was designed to effectively leverage a smaller amount.
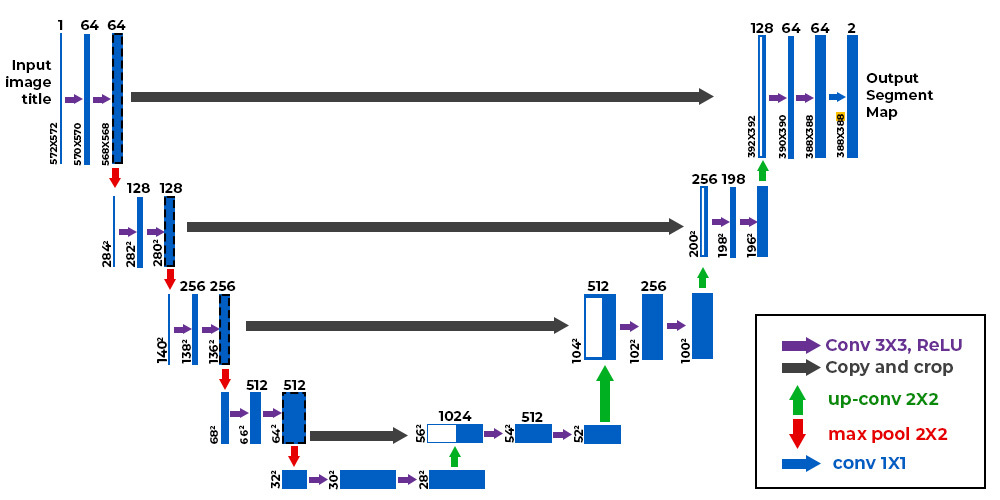
U Net Architecture Explained Geeksforgeeks U net. u net is an architecture for semantic segmentation. it consists of a contracting path and an expansive path. the contracting path follows the typical architecture of a convolutional network. it consists of the repeated application of two 3x3 convolutions (unpadded convolutions), each followed by a rectified linear unit (relu) and a 2x2. This is a defining feature of u net. u net is an encoder decoder segmentation network with skip connections. image by the author. u net has two defining qualities: an encoder decoder network that extract more general features the deeper it goes. a skip connection that reintroduces detailed features into the decoder. The u net is composed of two main components: a contracting path and an expanding path. contracting path : aims to decrease the spatial dimensions of the image, while also capturing relevant. Figure 8: u net architecture (source: author) this is done by concatenating the last layer in the convolutional block and the first layer of the opposite deconvolutional block. the u net is symmetrical — the dimensions of the opposite layers will be the same. as seen in figure 9, this makes it easy to combine the layers into a single tensor.
Comments are closed.